Understanding the Impact of Customer Data on Predicting Trends and Refining Sales Tactics
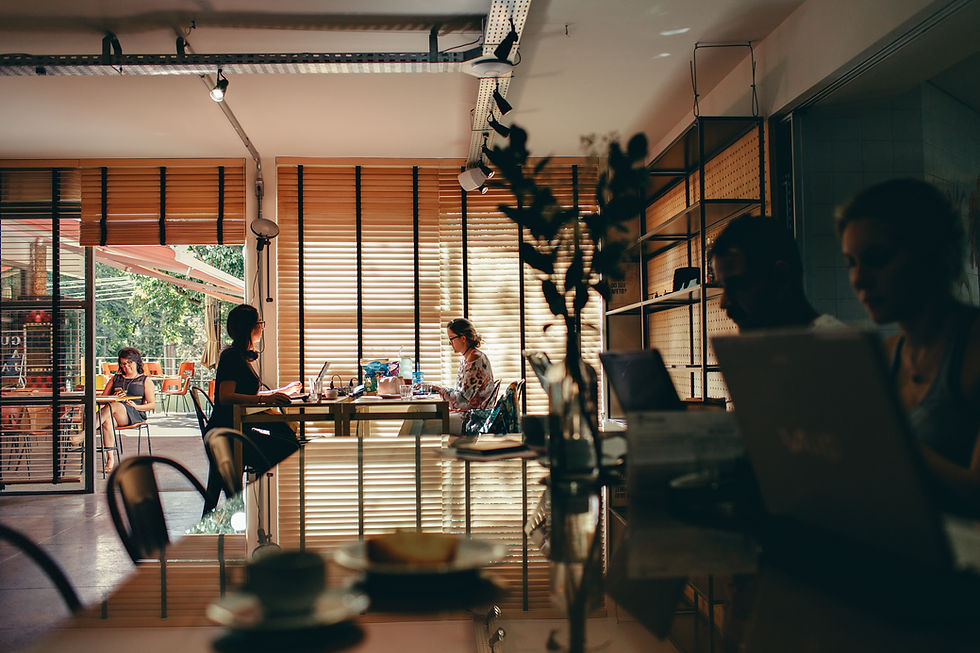
Sales forecasting has long been a critical tool for businesses, providing the insights needed to align resources, anticipate demand, and strategize effectively. The emergence of advanced data analytics, especially around customer behavior, is reshaping this landscape.
As organizations move towards data-driven decision-making, the use of customer data analytics has become integral to refining sales forecasts, improving accuracy, and predicting trends. This article explores how businesses can leverage these tools to gain more precise insights into customer behavior and ultimately drive better sales strategies.
Revolutionize Your Ad Campaigns! Are you tired of constantly worrying about your ad budget? Check out our monthly ad subscription plan. Learn more
The Shift to Data-Driven Sales Forecasting
In the past, sales forecasting often relied heavily on historical data and the intuition of sales teams. However, these traditional methods are proving less reliable in today’s rapidly changing markets.
With access to real-time customer data, companies are now able to analyze not only what customers have bought in the past but also predict future purchasing patterns based on behavior and interactions.
According to a study by McKinsey, organizations that utilize advanced analytics for sales decisions see an average improvement in sales performance by 15-20%. This improvement comes from better forecasting accuracy, which helps businesses adjust their inventory, target the right customers, and optimize marketing spend.
Understanding Customer Behavior Through Analytics
Customer data analytics goes beyond simply tracking sales figures. It involves gathering and analyzing diverse data sets such as browsing behavior, social media interactions, and purchasing history to paint a comprehensive picture of customer preferences. For example, a company using predictive analytics may analyze past purchasing patterns along with social media engagement to forecast future demand for specific products.
Businesses leveraging such insights can make informed decisions about when and how to engage customers. This includes identifying key moments when potential buyers are more likely to convert, as well as tailoring marketing efforts to their preferences.
According to a 2023 Deloitte report, companies utilizing data analytics to understand their customers' behaviors reported a 67% increase in lead generation compared to those using traditional methods . These results show the clear benefit of using customer data to enhance sales forecasting.
How Customer Data Improves Forecast Accuracy
One of the key advantages of customer data analytics is its ability to refine sales forecasts by predicting trends and identifying potential disruptions. For instance, real-time data feeds can highlight unexpected spikes or drops in customer interest, allowing businesses to adapt their strategies proactively.
Additionally, by combining customer data with external factors such as economic indicators or market conditions, businesses can develop more comprehensive forecasting models.
This shift has been particularly evident in industries with rapidly changing consumer behaviors, such as retail and technology, where customer sentiment can change quickly based on external influences.
Companies that successfully integrate customer analytics into their sales forecasting models can anticipate these changes and adjust accordingly, leading to better performance overall.
Integration of Data Analytics into Sales Strategies
As more companies recognize the value of customer data, integrating data analytics into their sales strategies is becoming essential. With the growth of e-commerce and online shopping, digital data streams provide actionable insights that were previously unavailable.
Businesses can now use data not only to forecast sales but also to optimize their customer engagement strategies. For example, by analyzing purchase patterns, companies can design targeted marketing campaigns aimed at high-potential customers, driving conversions and improving sales figures.
Implementing data-driven forecasting also allows companies to refine their pricing strategies, better manage inventory, and reduce unnecessary expenses. By adjusting supply chains and promotional efforts in real time, businesses avoid overstocking or stockouts, which can have significant financial consequences.
The Importance of Data Quality
While data analytics can revolutionize sales forecasting, it is essential to ensure the data being used is accurate and reliable. Poor-quality data can lead to faulty predictions, undermining the entire forecasting process. To combat this, companies must establish robust data governance practices, which include data cleansing, validation, and ongoing monitoring.
Research from the Harvard Business Review found that 47% of companies struggle with data quality issues, which negatively impact their ability to forecast sales . Businesses need to invest in technologies and processes that ensure clean and usable data to truly benefit from analytics.
Customer data analytics is fundamentally transforming sales forecasting by offering more accurate predictions and deeper insights into consumer behavior. By harnessing the power of customer data, businesses can better anticipate market trends, refine their strategies, and enhance overall sales performance. As the importance of data-driven decision-making continues to grow, businesses that embrace this shift will be well-positioned to stay ahead of the competition.
Comments